This workshop is organized with the support of RT-MIA, ANR PostProdLeap, MAP5, IMB.
Location
The workshop will be hosted at the
Centre Culturel Irlandais
5 Rue des Irlandais
75005 Paris
The CCI is accessible by bus or metro. (RER B “Luxembourg”, or Metro 7 “Place Monge” or “Cardinal Lemoine”).
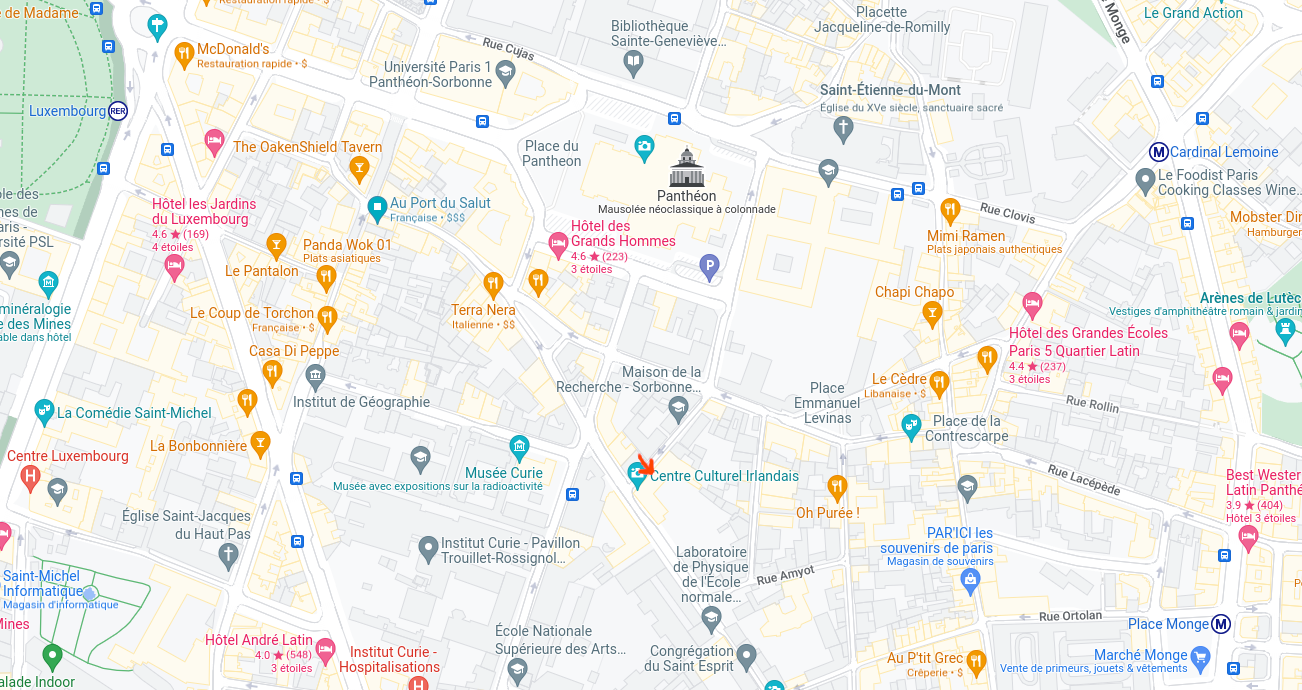
Abstract
Designing efficient algorithms for ill-posed inverse problems in imaging science crucially relies on the choice of a prior on the solution. It has been a long-standing issue to design regularity priors that allow to use optimization algorithms having good convergence guarantees and producing sharp visual results. The Plug-and-Play framework implicitly regularizes inverse problems in optimization algorithms by substituting the regularizer, its gradient or its proximal operator by learned representations thereof. State-of-the-art restoration results have been obtained with denoisers parameterized by deep neural networks, and by generative models such as GANs, VAEs, Normalizing Flows or diffusion models. More recently the PnP framework has also been exploited in Bayesian imaging where it has been succesfully applied to MMSE estimation, uncertainty quantification among other Bayesian computations. However, these successes raise many issues related to the convergence of these schemes or their recovery guarantees. Moreover, the design of accurate denoising neural network architecture or the tuning of the hyperparameters involved are open problems that strongly affect the PnP restoration performance.
The aim of this workshop is to gather leading experts of Plug-and-Play image restoration in order to discuss recent advances on these topics and to draw new collaborations to tackle the currently open questions.
Topics
- Deep regularizers
- Non-convex optimization
- Automatic parameter tuning
- Neural network architectures
- Recovery guarantees
- Deep unfolding
Replay the PnP workshop
Some of the presentations given in the workshop are available here.
Invited Speakers
- Regev Cohen (Verily Research), remotely
- Julie Delon (MAP5, Université de Paris)
- Johannes Hertrich (Institut für Mathematik, Technische Universität Berlin)
- Zahra Kadkhodaie (Center for Data Science, New York University), remotely
- Ulugbek Kamilov (Computational Imaging Group, Washington University)
- Marcelo Pereyra (Institute for Mathematical Sciences & Heriot-Watt University)
- Matthieu Terris (Heriot-Watt University)
- Kai Zhang (Computer Vision Lab, ETH Zurich)
Detailed Schedule
The detailed schedule including abstracts is available here
Please note that the time slots are expressed in local time in France (UTC+1).
Wednesday December 7th
- 8:45am-9am: Welcome of participants
- 9am-9:15am : Workshop opening
- 9:15am-10am Tutorial session : Samuel Hurault (Institut de Mathématiques de Bordeaux)
Introduction to Plug-and-Play methods and to their convergence analysis - 10am - 10:45am : Regev Cohen (Verily Israel, remotely)
The Fixed Points of Plug-and-Play Methods - 10:45am - 11:15am : Break
- 11:15am - 11:40am : Sebastian Neumayer (EPFL Lausanne)
Convex Regularizers based on Shallow Neural Networks - 11:40am - 12:05pm : Rita Fermanian (Inria Rennes)
PnP-ReG: Learned Regularizing Gradient for Plug-and-Play Gradient Descent
- 1:35pm - 2:20pm : Johannes Hertrich (TU Berlin)
Convolutional Proximal Neural Networks and Plug-and-Play Algorithms - 2:20pm - 3:05pm : Matthieu Terris (Heriot-Watt University)
Plug-and-play algorithms through the lens of monotone operators - 3:05pm - 3:30pm : Jonathan Chirinos Rodriguez (University of Genova)
Learning Firmly Nonexpansive operators - 3:30pm - 4pm : Break
- 4pm - 4:45pm : Kai Zhang (ETH Zurich)
Deep Plug-and-Play and Deep Unfolding Methods for Image Restoration - 4:45pm - 5:10pm : Alban Gossard (Institut de Mathématiques de Toulouse)
Training adaptive reconstruction networks for inverse problems and applications to blind inverse problems - 5:10pm - 5:35pm : Valentin Debarnot (Basel University)
Deep-Blur: Blind Identification and Deblurring with Convolutional Neural Networks - 5:35pm - 6pm : Charles Laroche (GoPro & MAP5, Université Paris Cité)
Deep Model-Based Super-Resolution with Non-uniform Blur
Thursday December 8th
- 9am - 9:45am : Julie Delon (MAP5, Université Paris Cité)
PnP sampling for inverse problems in imaging - 9:45am - 10:30am : Marcelo Pereyra (Institute for Mathematical Sciences & Heriot-Watt University)
Bayesian inference with learnt generative image priors encoded by neural networks - 10:30am - 11am : Break
- 11am - 11:25am : Jean Prost (Institut de Mathématiques de Bordeaux)
Diverse super-resolution with pretrained hierarchical Variational Autoencoders - 11:25am - 11:50am : Charlesquin Kemajou (Heriot-Watt University)
Maximum marginal likelihood estimation of regularisation parameters in Plug-and-Play Bayesian estimation
- 2pm - 2:45pm : Ulugbek Kamilov (Washington University in St Louis)
Plug-and-Play Models for Large-Scale Computational Imaging - 2:45pm - 3:30pm : Zahra Kadkhodaie (New York University)
Stochastic Solutions for Linear Inverse Problems using the Prior Implicit in a Denoiser - 3:30pm - 4pm : Break
- 4pm - 4:25pm : Christophe Kervazo (Télécom Paris)
Unrolling PALM for sparse semi-blind source separation - 4:25pm - 4:50pm : Maud Biquard (ISAE-SUPAERO & CNES)
Deep regularization for inverse problems in satellite imaging - 4:50pm - 5:15pm : Vasiliki Stergiopoulou (Université Côte d’Azur)
PnP COL0RME : Fluorescence image deconvolution microscopy using a Plug-and-Play Denoiser - 5:15pm - 6pm : Tutorial session : Valentin De Bortoli (CNRS, ENS Ulm)
Denoising diffusion models for inverse problems